How can you perform A/B testing on your ads?
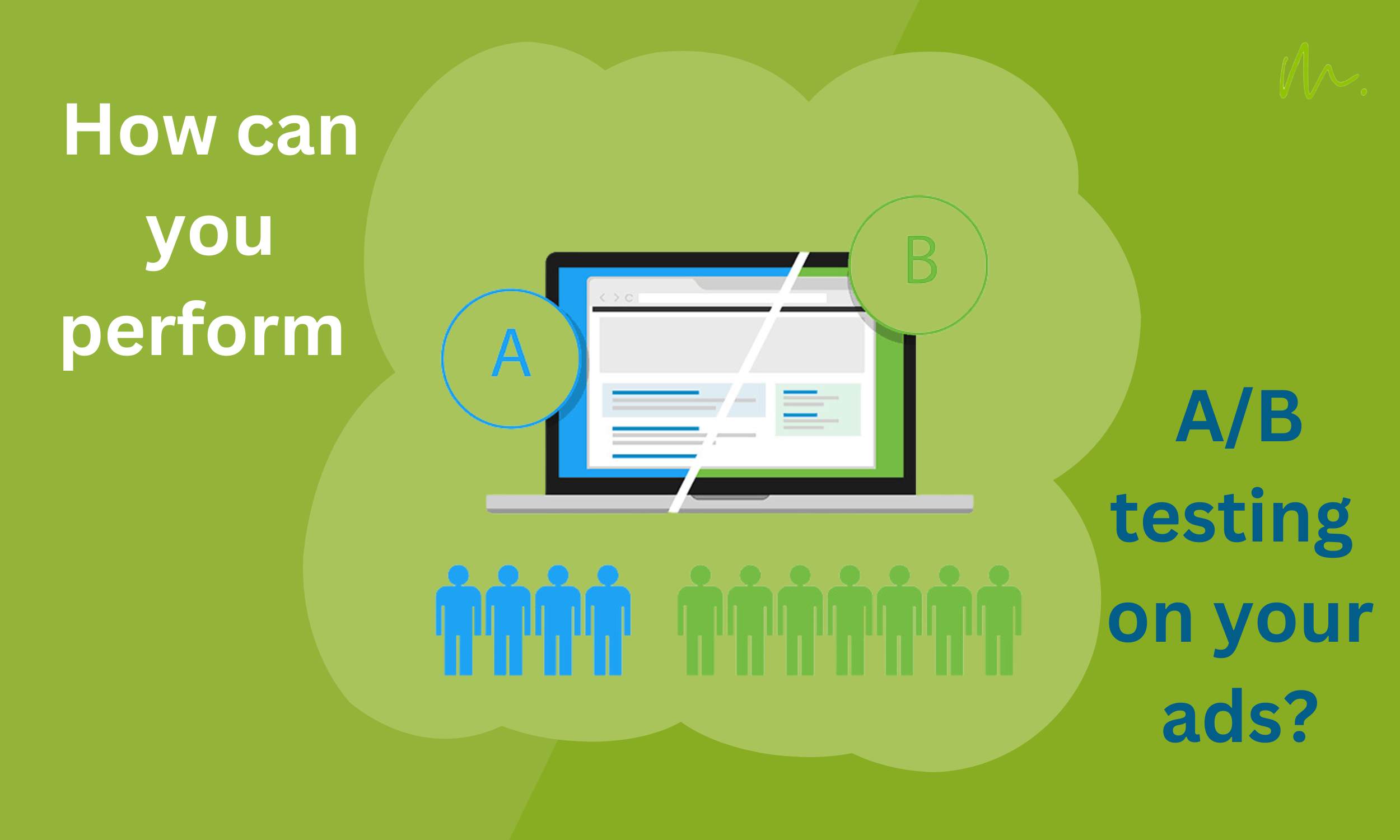
Are you aware of a technique which is rather effective when it comes to advertisement is A/B test or split test that helps compare two versions of advertisement. In this process the elements in the ads are changed and one can study the impact of the target audience’s response.The A/B testing can help you improve your ad performance, increase conversions, and maximize your return on investment (ROI). So, in this blog, you will learn how you can carry out A/B testing for your ads properly.
Table of Content –
- What is A/B testing?
- Purpose of A/B testing on ads
- How to run an A/B test on ads
- Understanding A/B test results
- Best practices of A/B testing
- Conclusion
- FAQs
What is A/B testing?
A/B testing or split testing is a process of comparing two or more variations of a web page, email, ad or other marketing communication tool in order to define which one yields better results. The purpose is to identify changes that can increase conversion rates, engagement or other key performance metrics.
A/B testing is a powerful tool for data-driven decision-making which allows businesses to optimize their marketing strategies and improve user experiences.
Purpose of A/B testing on ads
🎯 Optimizing Performance: By comparing two versions of an ad, the marketer is able to understand which one is more effective in terms of appealing to the target demographic and garnering more attention and website traffic, CTR, and conversions.
🎯 Understanding Audience Preferences: A/B testing enables us to identify which parts among the headlines, visuals, copy, CTAs, or landing pages grab the audience’s attention more. This insight can be used in the next sequence of ads and the message to be conveyed to the public.
🎯 Improving ROI: Thus, it is possible to guarantee that by knowing the best ad variation, businesses can effectively manage their budget and get the maximum ROI from the ad spend.
🎯 Data-Driven Decision Making: Bit A/B testing gives the best proof of what factors are effective or ineffective. This eliminates guesswork and makes it easier to follow real data in the placing of ads and choice of campaigns.
🎯 Reducing Risk: By trying out various versions, marketers are guaranteed to avoid the possible misfortune of a company launching a less successful campaign in a bigger market setting.
🎯 Incremental Improvements: Similar to all forms of online marketing, A/B testing can be performed repeatedly on a regular basis in order to enhance the ads hence the marked progressive improvement in the overall efficiency of the ads.
How to run an A/B testing on ads?
👉 Define Your Objective
Decide the goal of your A/B test. This could be to increase click-through rates (CTR), conversions, engagement or any other measurable metric.
👉 Identify the Variable to Test
It is advised to choose only one element at a time to vary in order to enhance the clarity of results. This could be:
- Creative elements: Images, videos, or graphics.
- Text: Headlines, ad copy, or calls-to-action.
- Targeting: Audience segments, demographics, or interests.
- Ad placement: Where the ad appears (e.g., social media platforms, search engines).
- Landing pages: The page users land on after clicking the ad.
👉 Create the Variants
Develop two versions of the ad: namely the original and the variation. Make sure that the only variation is the component under investigation being present in one and absent in the other.
👉 Set Up the Test
It may be also useful to select an ad platform that supports A/B testing such as Google Ads, or Facebook Ads Manager. Develop a campaign and make sure that both versions are displayed to the same target audience at the same time.
👉 Allocate Your Budget
Split the money into two halves whereby each half is given to one of the two ad variants. This makes it possible for each of the ads to get a fair run at being displayed and in gathering data.
👉 Run the Test
Place the ads and let the test run for a long enough time to collect enough data. The time depends on the traffic and the level of statistical significance if one wants to attain. Such a period may take between a couple of days and a couple of weeks.
👉 Monitor Performance
Monitor the performance of the test towards the key metrics that you have established for the test. This includes CTR, Conversion rate, CPA among others Ad platforms and come with analytic tools that are useful in tracking these parameters.
Understanding A/B testing results
Let us discuss A/B testing results with an example –
Suppose you run an online store and want to test two versions of a Facebook ad to see which one generates more clicks.
- Control (Ad A): Original version with a blue background and the headline “Shop Our New Summer Collection!”
- Variation (Ad B): Test version with a green background and the headline “Discover Your Summer Style!”
Metrics Tracked:
- Impressions: The number of times the ad was shown.
- Clicks: The number of times users clicked on the ad.
- Click-Through Rate (CTR): The percentage of impressions that resulted in clicks, calculated as (Clicks/Impressions) * 100.
Results:
- Ad A (Control):
Impressions: 10,000
Clicks: 200
CTR: (200 / 10,000) * 100 = 2%
- Ad B (Variation):
Impressions: 10,000
Clicks: 300
CTR: (300 / 10,000) * 100 = 3%
Analysis:
- CTR Comparison: Comparing CTR values, Ad B has a greater click through rate of 3% against the click through rate of ad A which is 2%.
- Statistical Significance: The next condition is that their difference should be statistically significant to conclude that Ad B is better indeed. This can be computed using a statistical significance calculator or other related tool that would use the small sample size and variance.
Conclusion:
- You can conclude that the green background and headline “Discover Your Summer Style!” in getting better clicks.
- As a result, you may decide to use Ad B as your main ad and potentially explore other variations to optimize further.
Best Practices of A/B testing
✅ Test One Variable at a Time: To correlate the observed behavioral changes with a specific element in order to see the results.
✅ Ensure Statistical Significance: Do not be quick to jump to different conclusions when you do not have enough information that can point to that conclusion.
✅ Keep External Factors Constant: As much as can be, influence other factors (independent variables such as day time and target audience) to be at their best to affect a valid test.
✅ Use a Large Enough Sample Size: It is also important that each ad variant is targeted to a relevant sample size so that the results are statistically significant for each set of ads.
Conclusion
A/B testing thus becomes an informative tool for the advertisers in the analysis of their campaigns. Indeed, when each component is tested independently and changes are based on statistical findings then your advertisements will be more effective to attain your marketing objectives. Thus, A/B testing should be seen as a long-term process and constant fine-tuning is required to maintain success in the constantly shifting online world.
Start experimenting with the A/B testing on ads with TheManinderShow where we help our clients to improve their ad performance.
FAQs –
Q) How do I choose what to test in an A/B test?
Ans. Begin by determining the external variables which influence the ads’ performance and are in harmony with the specified goals. Initially, it is recommended to test the key areas that are the headline and CTA while making sure that only one change is made at a time in order to examine its effectiveness.
Q) How long should an A/B test run?
Ans. The duration of an A/B test depends on your traffic volume and the time needed to gather statistically significant data. It is often wise to let the test run for as long as possible, minimally one week, but it depends on the circumstances.
Q) Can A/B testing be used on other marketing channels besides ads?
Ans. Of course, it is possible to adopt A/B testing for other marketing channels which include emails, landing pages, website and social media accounts, posts. The principles remain the same: compare and contrast in relation to a variable.
Recent Comments